When Big Data Doesn't Translate

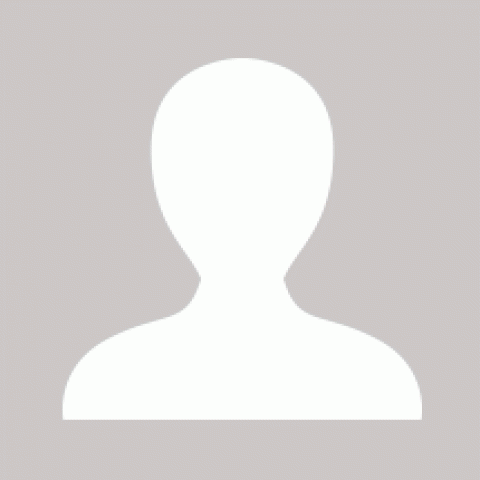
0 min read
Zack Rutherford
Big Data is more than just a giant buzzword. The enormous effort of gathering and interpreting billions upon billions of bits of data is revolutionizing the tech industry as a whole and it’s doing a number on the eCommerce world in particular. The first, most pressing problem with Big Data is everyone is pretty good at getting it, but not so good at figuring out what to do with it once they’ve got it.
The advantages of Big Data are touted loudly and often.
Volume—An enormous amount of information that can help you glean valuable business insightsVelocity—Proper and effective data analysis will allow you to enact change to your business in real time.Variety—The different types of data you can collect from various sources all combine to give you a broad and comprehensive view of what your customers want and how they interact with your web presence.
Online retailers use the massive amount of diverse data delivered at disproportionate speeds to personalize their offers to customers, improve customer service, alter their pricing strategies in real-time, predict shifts in their market, and keep better track of their supply chains. It can even be used to identify loss prevention opportunities such as fraud or scamming. In sum, it’s a very powerful tool so long as it’s used effectively and wisely.
What doesn’t get as much air-time as the benefits, however, are the various pitfalls that invariably come up while implementing a Big Data strategy. Today, we’ll take a look at some of the keys to properly applying Big Data insights to your eCommerce business, as well as a few of the reasons why more information doesn’t always equal better practices.
Big Data Background
Before we dig deep into the problems associated with analysis and implementation, let’s take a quick moment to review the different types of data we’ll be discussing.
Different Big Data Sources and Uses in eCommerce There are many different types of data that are collected by online retailers that have a direct effect on their big data initiatives. Many of these are sales and marketing figures such as:
Number of orders Products viewed Shopping cart abandonment rate Unique visits as a result of a marketing campaign Traffic resulting from referral links Data gained from cookies/remarketing Browsing behavior observed via Google Analytics or another analytics tool
More specifically, these different sources provide retailers with actionable data. This is data that can be used in order to make positive changes to an e-tailer’s design, business model, sales funnel, customer service, or even their branding efforts. Such data that many eCommerce sites highly value would include facts and figures that would aid in processes.
Stats like:
Collaborative filtering /Product recommendations Indicating trends or lack of trends Anticipating future popularity of products and promotions Measuring marketing outcomes against sales or the number of customers Making associations between on-page elements or keywords, and the specific link chosen Segmentation
As you can tell, the manifold types of data certainly have no lack of utility. Unfortunately, many Big Data projects never fulfill expectations. According to a study performed by Pricewaterhouse Coopers and Iron Mountain, a staggering 43% of companies realize little actual benefit from their Big Data projects and a further 23% fail to realize any benefit at all.
The problem is essentially one of signal versus noise. The very advantages of mass data collection can also be seen as major challenges. Because you have all of this diverse, disparate, and frankly overwhelming data at your disposal, it can be challenging to isolate the important jewels of information from the dross. For example, how does the ratio between the number of clicks per page from an individual user in Peru versus those from an individual user in Arkansas really affect your overall business model?
It doesn’t. So don’t bother to segment your metrics that way. This may seem a humorous aside, but it actually leads us to our first important point about the reasons a Big Data project might fail.
Data Train off The Rails
A lack of clear goals from the organizational leadership will make it impossible for your data analysts to separate the signal from the noise. Noted author and statistics expert, Nate Silver outlined a number of major missteps that many big companies make when working with Big Data in a speech at the 2015 Rich Data Summit.
According to Nate, the idea that massive datasets automatically equal valuable business insights is not only mistaken, but also quite dangerous. Namely because more data doesn’t mean better interpretation. Silver pointed to politicians as repeat offenders of bringing up data to support their preconceived biases when debating issues. Meanwhile, the most effective method of interpretation is to do the exact opposite, that is, to start with no hypotheses and see what the data says without bias.
When it comes to eCommerce, the same sort of pitfalls are present. Company leadership can often draw false conclusions from large volumes of data. Seeing what they’d like to see, rather than the actuality of the situation. This is due to the basic human instinct of pattern recognition. As an evolutionary imperative, we look for patterns in systems in order to predict outcomes and exploit the systems to our benefit.
The trouble is, we can sometimes fool ourselves into believing the noise is actually the signal. This is doubly true when parsing the enormous volumes of data represented in business analytics. The three main obstacles to drawing useful conclusions from big data are:
Efficiency Implementation And clear, concise direction
Efficiency
The difficulty is in the timing. Big Data is often very time sensitive. This means that your analysis and filtering between signal and noise is going to be high pressure and top heavy. You’ll need to get a lot of difficult work done in a relatively short time frame.
In order to cope, you’ll have to develop effective systems for analysis and using reliable tools for collection and filtering. We’ll talk more about the analysis in the next section, but for now let’s concentrate on which tools you can use to make your data journey a little easier.
The Google Analytics system helps you generate highly specified reports and is easily integrated with all the major eCommerce platforms. There’s a steep learning curve, but plenty of educational resources are out there.
Kissmetrics
KissMetrics tracks users who visit and purchase giving you a ton of extremely useful browsing information over multiple sessions. Very easy to organize the data and integrates well with optimization and a/b testing tools as well.
CrazyEgg
Heatmaps. See where your users click, hover, and interact. CrazyEgg segments users by source and tracks their actions so you can see which of your users are the most engaged. It’s an absolutely essential tool for optimization and data collection.
Exponea
Exponea actually takes the data it collects and uses it to automate marketing efforts. A truly robust turnkey analytics solution, Exponea collects a mass of diverse data about your users and their interactions on your site. Most impressively, it designs email, SMS, and push notification campaigns based on the data that it collects.
Implementation
It’s one thing to collect a ton of information, but another to put it to good use. The difficulty here is there’s a dearth of professionals who are qualified to analyze and interpret data. Data scientists and analysts are in high demand and finding a competent employee that can distill your data into actionable insights can be a major benefit to your business.
Unfortunately, the competition to fill a data science position is cutthroat. According to a study by RJmetrics, demand for data scientists is approximately double the supply. While more data scientists are graduating from colleges every year, you’ll have to have a sizable budget to afford them. The national median is around $119,000/year.
Assuming that the average eCommerce SMB may not have that kind of capital in the piggy bank, there are options for ad-hoc education. In general though, there are no shortcuts. You’ve got to learn algorithms, linear algebra, statistics, and myriad other skills that take a significant amount of time.
Sorry I don’t have better news. But if you’re interested in improving your skills one step at a time, here are some resources to get you started:
On the other hand, there are some data savvy entrepreneurs who are offering their expertise as a service. You can find and employ such services for a limited time while you gain your sea legs.
Direction
The final obstacle you’ll need to conquer is organizational direction. You’ve got to know what your goals are for your big data efforts. Remember that data collection is only useful so long as it meets a need. You have to define your business goals and collect data which translates to actionable insight.
Moreover, you need to define your endpoint for each project. Without a tangible objective, you can collect data forever without gaining anything from it. As we’ve discussed data science professionals don’t come cheap so this can be a major drain on your resources.
That about does it for our discussion on data. If you have any questions or observations, feel free to opine in the comment section below.
Images via Pixabay, Wikicommons, Kissmetrics, Crazyegg, Exponea